Elias Stengel-Eskin
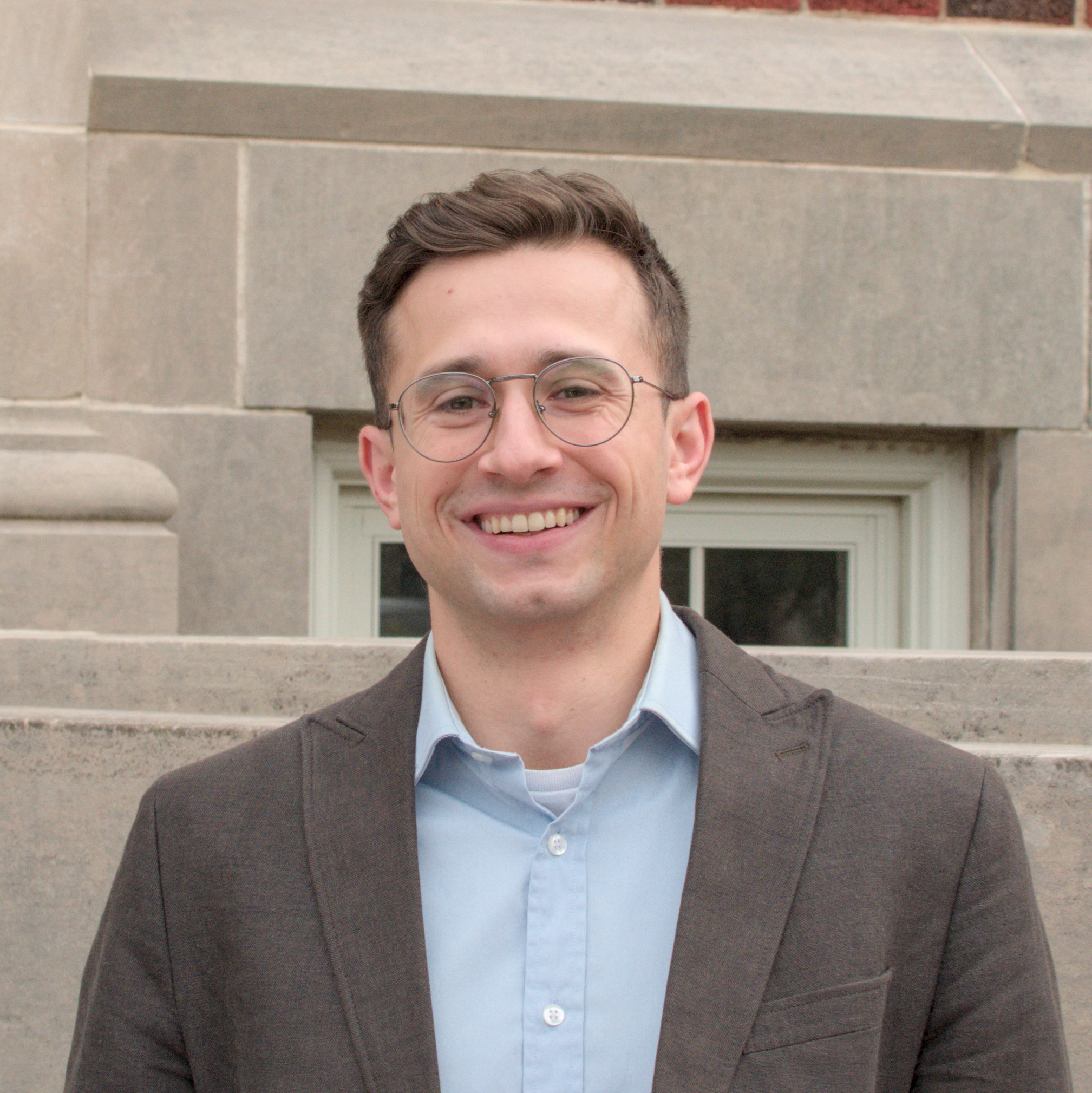
I am a Postdoctoral Research Associate at the University of North Carolina, Chapel Hill in the MURGe-Lab led by Mohit Bansal. I received my Ph.D. in 2023 from Johns Hopkins University, where I was supervised by Benjamin Van Durme and supported by an NSF GRFP.
I aim to develop AI agents that can intelligently communicate and collaborate with people and each other. My work addresses three key problems:
- A central focus of my work is multi-agent communication and collaboration, which has led to work on multi-LLM multi-round discussions/debates, distilling multi-agent behavior, pragmatic/verbalized uncertainty, and persuasion.
- Agents must be grounded to the world through their inputs and actions: another line of my work covers multimodal grounding and converting language to action through semantic parsing, text-to-code, and learning abstractions and skills.
- Developing safe and robust agents means handling uncertainty, ambiguity, and underspecification. As we scale up tasks, underspecification and ambiguity will become increasingly relevant, especially when predicting actions/grounding to the world. My work covers calibration and uncertainty especially in connection with implicit phenomena such as vagueness, underspecification, and ambiguity. While I’ve mostly explored these topics through a linguistic lens, I am interested in their importance to intelligence more broadly.
Concretely, some of the areas I’ve been publishing on recently are:
- Confidence Estimation and Calibration:
- Ambiguity and Underspecification:
- Multi-Agent/Multi-Model Reasoning:
- on multi-agent training to accept good and resist bad persuasion (Stengel-Eskin et al., NAACL 2025)
- on structured distillation to learn from multi-agent discussions (Chen et al., ICML 2024)
- on a new benchmark to assess game-theoretic abilities for LLM agents (Duan et al., NeurIPS 2024)
- on multi-agent iterative coarse-to-fine refinement for reasoning tasks (Chen et al., 2024)
- on using bandits to select instance-level reward models for LLM alignment (Nguyen et al., 2024)
- on combining 16 LLMs on 1 GPU via symbolic MoE (Chen et al., 2025)
- Learning Skills and Abstractions for Agents/Coding/Planning:
- on learning coding abstractions with LLMs (Stengel-Eskin et al., ICML 2024)
- on discovering skills for RL agents (Fu et al., ICML 2024)
- on distilling skills from teachers for LLM agents (Hashemzadeh et al., CoLLAs 2024)
- on hybrid and controllable System 1 and System 2 planning (Saha et al., ICLR 2025)
- on learning to generate unit tests for LLM debugging (Prasad et al., 2025)
- Improving Multimodal Models and LLM Agents:
- on building and testing data generation agents for creating training data (Khan et al., ICLR 2025 Spotlight)
- on a tree-based representation for LLM-based video reasoning (Wang et al. CVPR 2025)
- on improving visual prompting/object grounding without training (Wan et al., ECCV 2024)
- on a more effective/efficient self-consistency method for LLM agents (Wang et al., ACL 2024)
- on Western cultural bias in VLMs and the effect of pretraining language (Ananthram et al., ICLR 2025)
- on visual commonsense in unimodal and multimodal models (Zhang et al, NAACL 2022)
Before starting my Ph.D., I received my B.A.&Sc. with First Class Honours in Cognitive Science from McGill University, focusing in computer science and linguistics. While at McGill, I worked as a research assistant at the Montreal Language Modeling Lab (MLML), now MCQLL supervised by Morgan Sonderegger. I wrote my honours thesis (supervised by Timothy O’Donnell) on a variational inference algorithm for a model of language acquisition.
news
Feb 26, 2025 | VideoTree: Adaptive Tree-based Video Representation for LLM Reasoning on Long Videos accepted to CVPR 2025! VideoTree augments LLMs with tree structure for reasoning over long videos. |
---|---|
Feb 11, 2025 | DataEnvGym: Data Generation Agents in Teacher Environments with Student Feedback led by Zaid Khan with Jaemin Cho and Mohit Bansal has been selected as a ICLR 2025 Spotlight! |
Feb 7, 2025 | New preprint! Learning to Generate Unit Tests for Automated Debugging with Archiki Prasad, Justin Chih-Yao Chen, Zaid Khan, and Mohit Bansal on how to improve automated debugging with generated unit tests. We train unit test generators, leveraging test-time scaling and validation across tests to improve debugging! |
Jan 23, 2025 | Six new papers accepted, three to ICLR and three to NAACL! DataEnvGym (ICLR) introduces a new framework for developing agents that adaptively generate data for training student models. System 1.x (ICLR): planning with LLMs that balances quick action prediction with slower/more deliberate planning through verbalizing search traces. See It from My Perspective (ICLR) quantifies the effect of language on cultural bias in large vision-language models. Persuasion-Balanced Training (NAACL): multi-agent training method teaching models to balance accepting good persuasion while resisting misinformation/bad persuasion. AdaCAD (NAACL): an adaptive method for balancing retrieved/context knowledge with a model’s parametric knowledge. MAMM-Refine (NAACL) improves generation through multi-agent multi-model discussion, focusing on refinement. |
Nov 6, 2024 | Our philosophy collaboration on challenges in model editing was accepted to TMLR! Fundamental Problems With Model Editing: How Should Rational Belief Revision Work in LLMs? |
Oct 21, 2024 | New paper out! Teaching Models to Balance Resisting and Accepting Persuasion, where we use multi-agent recursive dialogue trees to teach models to accept and resist persuasion when appropriate. Our method reduces susceptibility to misinformation and flipflopping while also improving LLMs’ ability to act together in a team thru multi-agent dialogue! |
Oct 11, 2024 | New preprint! DataEnvGym: Data Generation Agents in Teacher Environments with Student Feedback led by Zaid Khan with Jaemin Cho and Mohit Bansal on a novel testbed for creating data generation agents. These agents produce synthetic data for teaching student models based on their errors and weaknesses! |
Oct 3, 2024 | New preprint! LASeR: Learning to Adaptively Select Reward Models with Multi-Armed Bandits led by Duy Nguyen and Archiki Prasad with Mohit Bansal on using bandit methods to pick the best-suited RM to optimize at an instance level, improving LLMs on reasoning, instruction-following, and long-context understanding. |
Oct 2, 2024 | Two papers accepted to NeurIPS 2024! LACIE: Listener-Aware Finetuning for Confidence Calibration in Large Language Models uses pragmatics to calibrate LLMs, and GTBench: Uncovering the Strategic Reasoning Limitations of LLMs via Game-Theoretic Evaluations introduces a new game-theoretic benchmark. |
Sep 19, 2024 | New preprint! MAgICoRe: Multi-Agent, Iterative, Coarse-to-Fine Refinement for Reasoning led by Justin Chih-Yao Chen with Swarnadeep Saha, Archiki Prasad, and Mohit Bansal introduces a novel method for refinement that improves math reasoning by selectively refining only hard instances and by treating it as an iterative, multi-agent problem. |
Sep 14, 2024 | New preprint! AdaCAD: Adaptively Decoding to Balance Conflicts between Contextual and Parametric Knowledge led by Han Wang with Archiki Prasad and Mohit Bansal introduces a dynamic decoding strategy to deal with variable amounts of knowledge conflict. |
Jul 1, 2024 | Contrastive Region Guidance: Improving Grounding in Vision-Language Models without Training has been accepted to ECCV 2024! |
Jun 3, 2024 | New preprint! LACIE: Listener-Aware Finetuning for Confidence Calibration in Large Language Models tackles implicit and explicit calibration in LLMs by using insights from pragmatics! |
May 28, 2024 | New project on videos+LLMs! VideoTree: Adaptive Tree-based Video Representation for LLM Reasoning on Long Videos uses a tree-based structure to help LLMs reason over long videos efficiently and effectively. Joint work with Ziyang Wang and Shoubin Yu. |
May 15, 2024 | Soft Self-Consistency Improves Language Model Agents has been accepted to ACL 2024! |
May 4, 2024 | Three papers accepted to ICML 2024! ReGAL: Refactoring Programs to Discover Generalizable Abstractions , which uses refactoring to discover program abstractions for LLM-based code generation, MAGDi: Structured Distillation of Multi-Agent Interaction Graphs Improves Reasoning in Smaller Language Models , which introduces a structured distillation method for learning from discussions between multiple LLMs, and Language-guided Skill Learning with Temporal Variational Inference, which learns reusable skills from trajectories of demonstrations. |
Mar 22, 2024 | Excited to be giving a keynote at the UncertaiNLP workshop at EACL 2024, titled Confidence-based Rephrasing, Refinement, and Selection. I’ll cover a wide range of topics including calibration in semantic parsing, using calibrated models to improve usability, underspecified visual question answering and much more! |
Mar 5, 2024 | New work with David Wan and Jaemin Cho on improving visual tasks (especially grounding) through region-based guidance in Contrastive Region Guidance: Improving Grounding in Vision-Language Models without Training |
Feb 3, 2024 | New work led by Justin Chen and Swarnadeep Saha on distilling multi-agent LLM interactions into smaller models: MAGDi: Structured Distillation of Multi-Agent Interaction Graphs Improves Reasoning in Smaller Language Models . MAGDi uses a graph structure on top of LLM dialogues to distill reasoning from several large teacher models into a single, lightweight student. |
Jan 30, 2024 | New preprint! ReGAL: Refactoring Programs to Discover Generalizable Abstractions introduces a new refactoring-based method for learning abstractions for LLM program prediction, improving performance on a variety of tasks. Joint work with Archiki Prasad as part of my postdoc at UNC. |
Jan 17, 2024 | Two papers accepted to ICLR 2024. Zero and Few-shot Semantic Parsing with Ambiguous Inputs introduces a new benchmark for semantic parsing with ambiguity and tests a variety of models on how they handle five common linguistic ambiguities. Rephrase, Augment, Reason: Visual Grounding of Questions for Vision-Language Models is the first paper from my new postdoc position and introduces RepARe, a method for augmenting and rephrasing VQA questions (especially underspecified ones) to make them easier for zero-shot VL models to answer. |
Jan 16, 2024 | My thesis is now publicly available: Modeling Meaning for Description and Interaction. Many thanks to my advisor Benjamin Van Durme for all of your guidance over the last five years and to my thesis committee Jacob Andreas and Kyle Rawlins for your feedback! |
Jun 3, 2023 | I’m incredibly excited to announce that I will be starting a Postdoc with Mohit Bansal at the University of North Carolina, Chapel Hill! Looking forward to lots of collaborations with the amazing students and faculty of UNC NLP and UNC CS! |
Jun 1, 2023 | Calibrated Interpretation: Confidence Estimation in Semantic Parsing has just been accepted to TACL! We examine the calibration of common semantic parsing models, including LLMs using in-context learning. Check out the paper for results across a number of tasks and datasets! |
May 3, 2023 | Why Did the Chicken Cross the Road? Rephrasing and Analyzing Ambiguous Questions in VQA has been accepted to ACL 2023! We introduce a brand new dataset of ambiguous questions in VQA, with a model disambiguation model and plenty of linguistic analysis. See you in Toronto! |
Mar 31, 2023 | I’ve restructured a previous pre-print into two different papers. The first focuses on cataloguing calibration in popular semantic parsing systems, and the second looks at what we can do with a well-calibrated model. |
Feb 28, 2023 | Super-CLEVR (CVPR highlight), an exciting new benchmark for generalization in vision tasks led by Zhuowan Li now accepted to CVPR 2023 as a highlight (~2% of submissions)! Super-CLEVR: A Virtual Benchmark to Diagnose Domain Robustness in Visual Reasoning |
Nov 30, 2022 | I am on the job market for faculty, postdoc, and industry positions! Please reach out if know of a role that would be a good fit for me: elias.stengel@gmail.com |
Nov 29, 2022 | Two new preprints out! On ambiguity in VQA and on calibration in semantic parsing |
Oct 7, 2022 | Two new papers accepted to EMNLP 2022. Preprints out on arxiv! On subject and object control in LLMs and on a troubling quirk in NLU |